Research in the lab covers a broad spectrum of multimedia signal processing and analysis. In recent years, researchers in the lab have pioneered the development of feature extraction with application to image registration, segmentation, steganography and information retrieval from large multimedia databases. The researchers in the lab participate in several interdisciplinary projects including the Bio-image informatics project whose goal is to develop, test and deploy a unique, fully operational distributed digital library of bio-molecular image data accessible to researchers around the world, and the graduate training program in Interactive Digital Multimedia.

High resolution imaging of molecules and cells will be critical for understanding complex systems such as the nervous system, whether it be for the localization of specific neuron types within a region of the central nervous system, the branching pattern of dendritic trees, or the localization of molecules at the subcellular levels. In collaboration with researchers in biology, we are developing new image analysis methods for quantifying complex biological phenomena. For more information about the Center for Bio-Image Informatics and the Bisque open-source system, click on the link above.
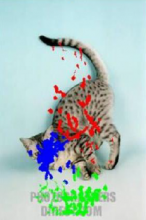
Humans are adept at complex visual tasks such as detection, tracking and recognition. The first aim of this project is to understand humans visual attention mechanism and the factors influencing it. In particular we investigate the importance of scene context in attention modeling. We have also developed human attention inspired models to build semantic priors for text detection which outperforms state-of-the-art. Additionally, we have also improved object detection and video object segmentation using eye tracking data.
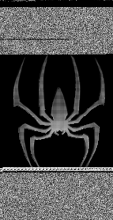
The goal of this project is to explore methods in Signal and Image processing for analyzing malware. Malware binaries are visualized as gray-scale images, with the observation that for many malware families, the images belonging to the same family appear very similar in layout and texture.
We exploit these visual similarities and dis-similarities and propose Image Similarity based features to the problems of Malware Classification, Detection, Retrieval and other areas.
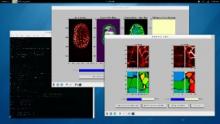
Robust methods for segmentation and tracking are critical for quantitative biology. We are developing methods for accurate, automated and interactive 2D/3D segmentation of cellular and sub-cellular structures, with emphasis on high-throughput image analysis. The three main issues that make analyzing microscopic imagery challenging are modeling of cell appearance which varies from one dataset to another, poor signal to noise ratio/clutter, and the large scale nature of the datasets. We have developed interactive static image segmentation methods using Markov Random Fields (MRFs) that utilize a human in the loop to achieve accurate segmentation. Further, we have proposed an approach for parameter adaptation that induces generic high-level priors on topology, facilitating robust tracing of neuronal structures. Recent work focuses on modeling edge appearance and scaling tracing techniques to hundreds of structures. Finally, we have also focused on the problem of detecting deformable structures such as synaptic junctions in Electron Micrographs.
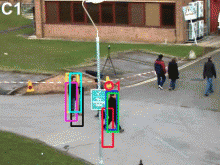
During the past few years we have set up a wide area camera network, called the SCALLOPSNet (Scalable Large Optical Sensor Network). About 100 stationary cameras covera an expansive area that can be divided into three distinct regions: inside a building, along urban paths, and in a remote natural reserve. Challenging research issues include geo-calibration, multicamera video summarization and anomaly detection, and multi-camera tracking and activity analysis in wide area networks.
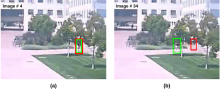
Most state-of-the-art object trackers assume good visual, clutter-free imaging conditions for reliable tracking. In real-life scenarios, encoding and transmission artifacts, object occlusions and scene clutter play major impediments for tracking performance. Humans on the other hand effortlessly track objects in such very challenging conditions. We aim to leverage human-generated knowledge to new challenging datasets for tracking objects.